Why Retail and Real Estate Analytics Are Mission-Critical in 2025
Jan 09, 2025 • 10 min read
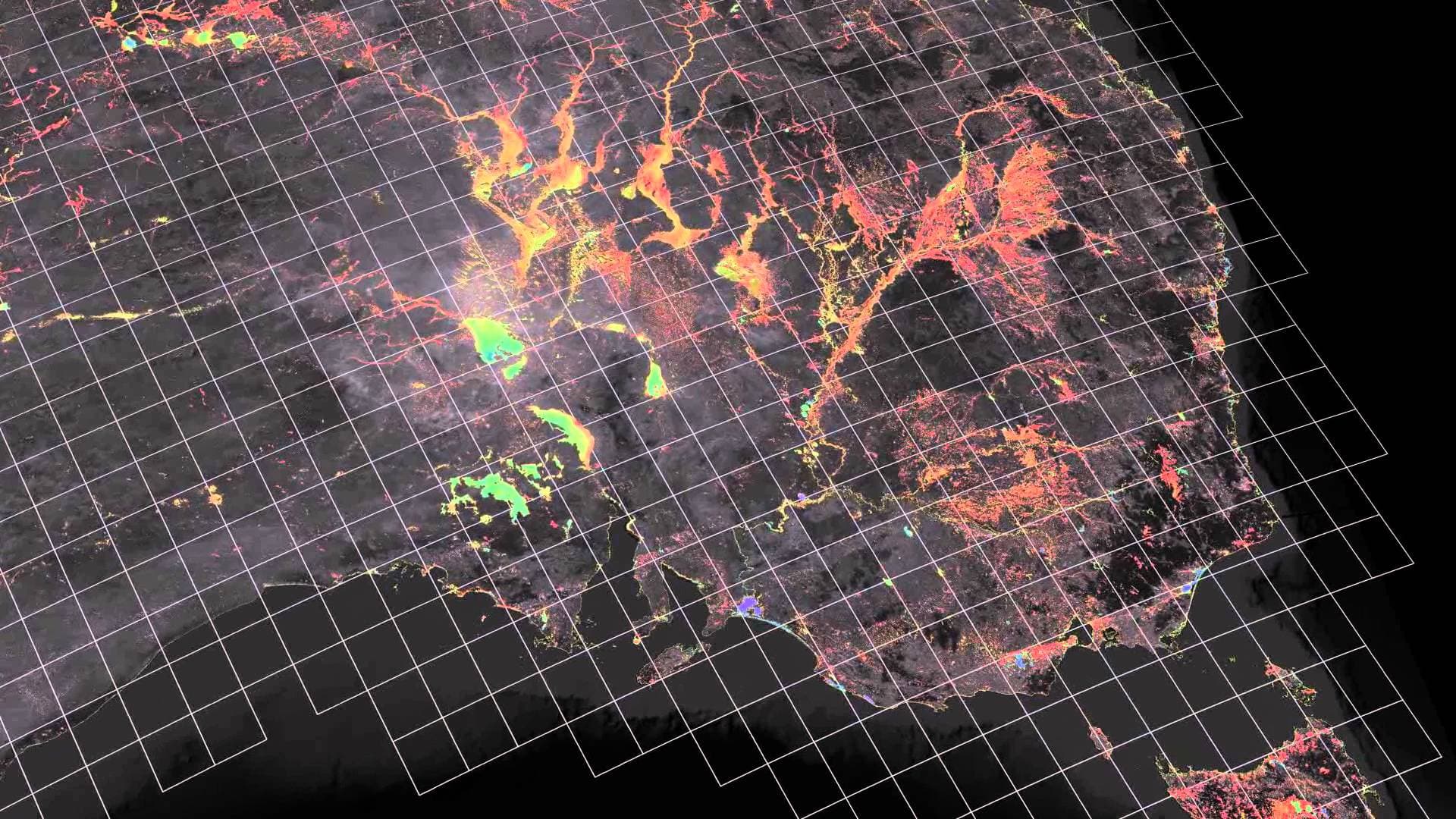
In today’s cost-crunched climate, owning or leasing commercial retail space has never felt so high-stakes. The last few years of rising interest rates, surging construction costs, and lukewarm consumer demand have conspired to squeeze margins across the commercial real estate (CRE) industry. As a result, landlords and retailers alike are turning to advanced analytics platforms to make real-time, data-driven decisions about who leases space, where, and on what terms. The risk of a bad bet—a suboptimal tenant or poorly chosen location—can mean the difference between stable cash flow and a downward spiral of vacancy and debt.
The Economic Pressures Driving Analytics Adoption
The economic backdrop for 2025 isn’t exactly gentle. Lending standards are more stringent, and interest rates hover near historic highs. A recent CBRE study showed that 79% of U.S. shopping center owners feel their net operating income is under severe pressure from escalating financing costs alone. Add in property taxes that have soared in many cities—up 12% on average since 2021, according to the National Council of Real Estate Investment Fiduciaries (NCREIF)—and it’s no wonder owners are desperate for tenants that promise steady, reliable foot traffic and rent payments.
On the flip side, retailers must navigate fierce competition and shifting consumer dynamics. A 2024 Deloitte survey found that 63% of retail executives rank “locational missteps” among the top three threats to profitability, underscoring the importance of precisely targeted site selection. With so little wiggle room in budgets, a single misallocated store can drag down an entire region’s performance.
“Margins have gotten so thin that we can’t afford a high-risk guess anymore,” says Olivia Chan, VP of Real Estate at an East Coast multi-brand retailer. “We look at everything—demographics, traffic patterns, competitor proximity—then run complex forecasting models before committing to a single new lease.”
The Rise of Retail Analytics Platforms
Retail analytics platforms have stepped onto the scene with promises to transform guesswork into science. Companies like Placer.ai, Buxton, and Near have attracted considerable funding by offering deep insights into shopper behavior—tracking everything from foot traffic and dwell times to psychographic data on local consumers. McKinsey’s 2025 ‘Retail Tech Landscape’ report notes that advanced analytics adoption among mid-sized chains has nearly doubled over the past two years, going from 22% to 41%.
“It’s an arms race for data-driven site selection,” says Craig Mercer, Senior Analyst at McKinsey. “At a time when ROI on new stores must be almost instant, operators need precise metrics—like how many potential customers walk past a location daily, the average spend profile in that zip code, and the presence (or absence) of competing concepts within a five-minute drive.”
These platforms integrate sales projections with local median incomes, mobile phone location data, and competitor footprints to yield what Mercer calls a “probabilistic forecast” of store success. The difference can be stark: a 2024 case study from a large apparel retailer found that using advanced geo-analytics cut the chain’s underperforming store ratio by 32%, an improvement worth tens of millions of dollars in saved overhead and better in-store sales.
Shopping Center Owners Turn to Property Intelligence
Meanwhile, on the landlord side, property intelligence tools—from CoStar to Reonomy and VTS—have evolved to provide robust tenant-mix recommendations and vacancy-risk assessments. A JLL survey from late 2024 found that 54% of shopping center owners now rely on predictive analytics to decide how to subdivide vacant big-box spaces or to forecast rent growth in newly repositioned centers.
“We essentially treat each prospective tenant as an investment prospect,” says Peter Rodriguez, Director of Leasing at a major REIT specializing in suburban retail properties. “We delve into their historical sales patterns, credit risk, online reviews, and forward-looking business plans. Then we cross-reference that with real-time shopper data in our submarket. We’ve eliminated more than half of our typical guesswork.”
Rodriguez points to an instance where analytics helped avert disaster: A struggling apparel chain offered top-dollar rent for a prime spot in a newly redeveloped center, but the REIT’s data forecasted plummeting foot traffic for that retailer’s entire store base. They turned down the chain in favor of a slightly lower-rent grocery concept that the data indicated would fare better. “Twelve months later, that apparel chain declared bankruptcy, closing dozens of stores,” he says. “Analytics saved us from a near-certain vacancy.”
Real-Time Data for Tighter Margins
The shift from quarterly or annual vantage points to near-real-time metrics is perhaps the most dramatic change. Tools that constantly refresh foot traffic counts, competitive openings, and local spending trends let both retailers and landlords make nimble decisions. If a store underperforms in its first six months, analytics can identify whether location, marketing, or merchandise mix is the culprit—enabling swift corrective action.
“We used to wait for monthly sales reports and aggregated foot traffic stats,” says Daniel Waters, CFO of a mid-sized convenience store chain. “Now, we can track daily footfalls against store-level labor spend and product distribution. Seeing a dip in morning coffee runs? We might adjust promotions on the fly or tweak the store layout to highlight a new breakfast offering. This data-based agility is a lifesaver in today’s environment.”
According to a 2025 RBC Capital Markets note, retailers who adopt real-time analytics see average store-level profit margins about 2–3 percentage points higher than those who rely on more static forecasting. For large chains operating hundreds or thousands of locations, those points can equate to hundreds of millions in additional net income.
Avoiding the Pitfalls of Over-Reliance
Of course, data can’t solve every problem. Over-reliance on analytics without on-the-ground insight can lead to false confidence. “If your data is incomplete or biased, you could choose a location with perfect metrics but hidden logistical nightmares—like a road construction project about to choke traffic for 18 months,” warns Jennifer Liu, Head of Research at UrbanStreet Advisors.
Experts recommend retailers and landlords combine quantitative models with local scouting and community feedback. Additionally, analytics should be continuously vetted. “We run real-world tests,” says Waters. “If the model predicts 1,200 daily footfalls, but we see only 900, we don’t just accept it—we revisit our assumptions, talk to our field teams, and refine the model accordingly.”
The 2025 Outlook
Despite economic headwinds, CRE deal flow in the retail sector hasn’t dried up—rather, it has become more selective. According to Colliers International, average cap rates for top-performing grocery-anchored centers remain stable, even as interest rates climb. Market watchers say that’s because investors trust the robust data fueling location decisions. Good analytics lead to better tenant curation, and better tenant curation underpins long-term rent stability.
“In 2025, the margin for error is razor-thin,” says Mercer of McKinsey. “Retailers can’t afford to pick a dud location, and landlords can’t afford to plug in the wrong tenant who fails in six months. Both sides rely on these analytics platforms, and the cost of not using them is only growing.”
Consequently, the future belongs to those who master retail and real estate analytics—embracing the marriage of big data with real-world nuance. For those who get it right, the payoff is fewer vacant store fronts, steadier rent rolls, and a consumer experience that matches what the local market genuinely needs. And in a landscape where every lease, every foot of retail space, and every shopper’s dollar is precious, that level of precision is no longer just a competitive edge—it’s survival.